360BEV: Panoramic Semantic Mapping for Indoor Bird's-Eye View
(WACV 2024)
Karlsruhe Institute of Technology
|
Hunan University
|
Zhejiang University
|
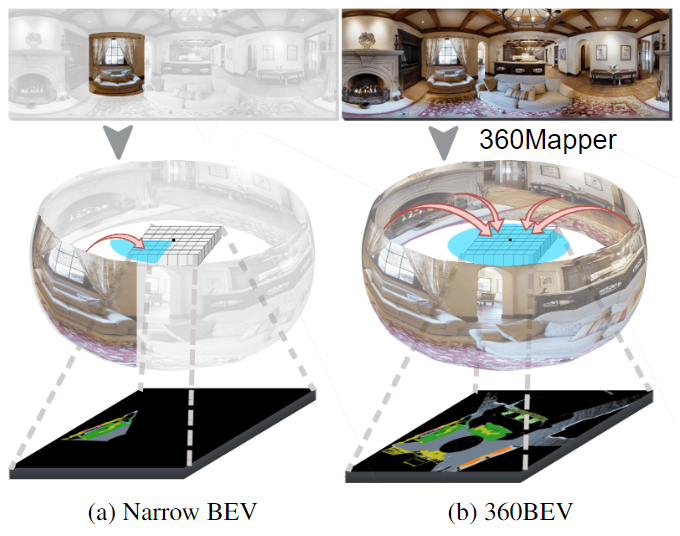
Fig. 1: Semantic mapping from egocentric front-view images to allocentric BEV semantics.
While (a) the narrow-BEV method has limited perception and map range,
(b) 360BEV has an omnidirectional Field of View, yielding a more complete BEV
map by using our 360Mapper model.
|
Abstract
Seeing only a tiny part of the whole is not knowing the full circumstance. Bird's-eye-view (BEV) perception, a process
of obtaining allocentric maps from egocentric views, is restricted when using a narrow Field of View (FoV) alone. In this
work, mapping from 360° panoramas to BEV semantics, the 360BEV task, is established for the first time
to achieve holistic representations of indoor scenes in a top-down view. Instead of relying on narrow-FoV image sequences,
a panoramic image with depth information is sufficient to generate a holistic BEV semantic map. To benchmark 360BEV, we
present two indoor datasets, 360BEV-Matterport and 360BEV-Stanford, both of which include egocentric panoramic images and
semantic segmentation labels, as well as allocentric semantic maps. Besides delving deep into different mapping paradigms,
we propose a dedicated solution for panoramic semantic mapping, namely 360Mapper. Through extensive experiments, our
methods achieve 44.32% and 45.78% in mIoU on both datasets respectively, surpassing previous counterparts with gains of +7.60% and +9.70% in mIoU.
360BEV Paradigms
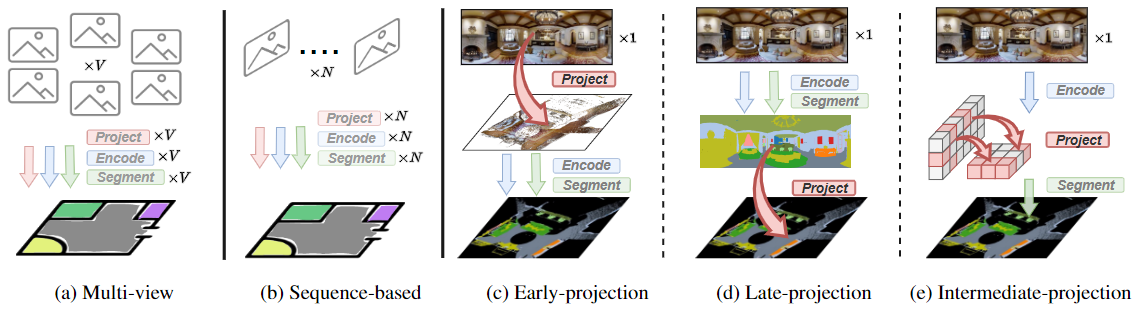
Fig. 2: Paradigms of semantic mapping. While the narrow-FoV (a) multi-view and (b) sequence-based methods rely on V≥6 and N≥20 views,
the 360°-BEV (c) Early-, (d) Late-, and (e) Intermediate-projection methods use a single panorama.
|
360BEV Dataset
Dataset statistic
360Mapper Model
Architecture of 360Mapper and the 360Attention module.
The 360Mapper model includes the encoder for extracting features
from the front-view panoramic image, the 360Attention module for feature projection,
and the decoder for parsing the projected feature to the BEV semantic map.
The offsets are obtained by a linear layer and added with the 2D index that
is obtained by Inverse Radial Projection (IRP), yielding the sampling
locations for 360BEV feature projection.
360Mapper Expeirmental Results
Results of panoramic semantic segmentation (360FV)
Results of panoramic semantic mapping (360BEV)
Per-class IoU results
Distribution of per-class semantic mapping results (per-class IoU in %)
on the 360BEV-Stanford and the 360BEV-Matterport datasets. On the 360BEV-Matterport
dataset, the results are compared on respective validation and test sets.
Compared to the baseline model Trans4Map, our 360Mapper models achieve overall better 360BEV results.
Visualization
360BEV-Stanford visualization
360BEV visualization and qualitative analysis on the 360BEV-Stanford dataset.
Black regions are the void class, indicating the invisible areas in BEV semantic maps.
360BEV-Matterport visualization
360BEV visualization and qualitative analysis on the 360BEV-Matterport dataset.
Black regions are the void class, indicating the invisible areas in BEV semantic maps.
Citation
If you find our work useful in your research, please cite:
@inproceedings{teng2023360bev,
title={360BEV: Panoramic Semantic Mapping for Indoor Bird's-Eye View},
author={Teng, Zhifeng and Zhang, Jiaming and Yang, Kailun and Peng, Kunyu and Shi, Hao and Reiß, Simon and Cao, Ke and Stiefelhagen, Rainer},
booktitle={WACV},
year={2024}
}
|